
AMETHYST: Design of polymer materials accelerated by artificial intelligence tools and high-throughput preparation and characterization methods
Coordinator: Jean-François GERARD
IMP – Ingénierie des Matériaux Polymères
(UMR 5223 CNRS/INSA Lyon/Univ. Jean Monnet St Etienne/Univ. Lyon 1)
Keywords: Polymer, High throughput, Artificial Intelligence
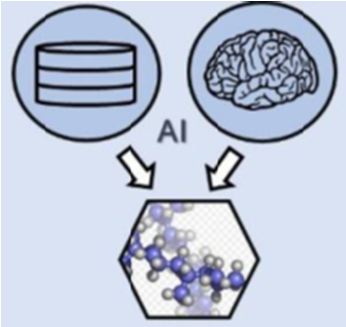
With global production of nearly 380 million tonnes per year, polymer materials play a central role in modern society. In fact, they are used in the manufacture of countless everyday products or as more sophisticated compounds in medicine, diagnostics and fine chemicals. However, economic constraints and new societal constraints require a more rational design and alternative modes of synthesis and formulation of polymers to meet the needs for greater sustainability, more virtuous end-of-life management and improved performance.
Polymer-based materials of the future will be one of the pillars of the circular economy. Thus, the discovery of new polymers will lead to a paradigm shift and new techniques for the design, processing and analysis of polymer-based materials. The recent development of high-throughput (HTP) and artificial intelligence (AI) methods has opened up enormous opportunities to address these challenges. Although such methods are emerging in chemistry, they are not yet implemented in France in Polymer Science. Thus, the AMETHYST demonstrator project proposes, as a proof of concept, to merge these fields through the combined use of HTP and AI methods to address four different case studies in the field of polymers. The issues addressed were chosen for their relevance to pressing scientific and societal issues:
- Case study 1 – Design of polymer materials with programmable degradability
- Case study 2 – Nanocomposites and polymer-based composites
- Case study 3 – Organic-inorganic materials based on polymers
- Case study 4 – Biosourced materials
In each case study, each type of material, method of synthesis and characterization of HTPs will be implemented at the molecular, macromolecular and materials scales. Data from the HTP analysis will be used to feed machine learning approaches to determine the best combination with multiple targeted properties. Optimized AI-designed materials will be manufactured and evaluated.